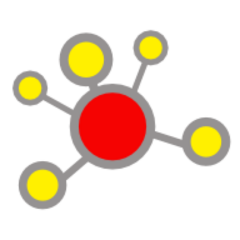
Centralize a graph according to the eigenvector centrality of vertices
Source:R/centralization.R
centr_eigen.Rd
See centralize()
for a summary of graph centralization.
Usage
centr_eigen(
graph,
directed = FALSE,
scale = deprecated(),
options = arpack_defaults(),
normalized = TRUE
)
Arguments
- graph
The input graph.
- directed
logical scalar, whether to use directed shortest paths for calculating eigenvector centrality.
- scale
Ignored. Computing eigenvector centralization requires normalized eigenvector centrality scores.
- options
This is passed to
eigen_centrality()
, the options for the ARPACK eigensolver.- normalized
Logical scalar. Whether to normalize the graph level centrality score by dividing by the theoretical maximum.
Value
A named list with the following components:
- vector
The node-level centrality scores.
- value
The corresponding eigenvalue.
- options
ARPACK options, see the return value of
eigen_centrality()
for details.- centralization
The graph level centrality index.
- theoretical_max
The same as above, the theoretical maximum centralization score for a graph with the same number of vertices.
See also
Other centralization related:
centr_betw()
,
centr_betw_tmax()
,
centr_clo()
,
centr_clo_tmax()
,
centr_degree()
,
centr_degree_tmax()
,
centr_eigen_tmax()
,
centralize()
Examples
# A BA graph is quite centralized
g <- sample_pa(1000, m = 4)
centr_degree(g)$centralization
#> [1] 0.1523145
centr_clo(g, mode = "all")$centralization
#> [1] 0.4128655
centr_betw(g, directed = FALSE)$centralization
#> [1] 0.2245963
centr_eigen(g, directed = FALSE)$centralization
#> [1] 0.9418167
# The most centralized graph according to eigenvector centrality
g0 <- make_graph(c(2, 1), n = 10, dir = FALSE)
g1 <- make_star(10, mode = "undirected")
centr_eigen(g0)$centralization
#> [1] 1
centr_eigen(g1)$centralization
#> [1] 0.75